Some great facts about neural networks
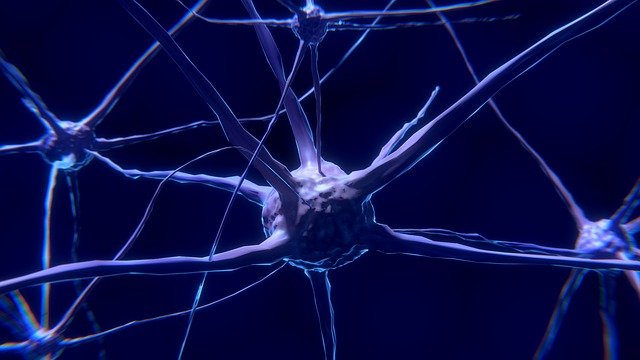
Regression models have been widely used for many years and have been extremely useful for modeling and making predictions of real-life situations in both science and manufacturing. At the same time, neural networks provide predictions close to the learning mechanism of the human brain even better than the supervised and unsupervised ML models.
This post discusses the pros and drawbacks of using neural networks for regression analysis.
Just what is the Regression Analysis?
Regression analysis will allow you to form the relationship between a dependent variable (which you try to predict) (the model’s output) and the input, which are the independent variables to be analyzed with some mathematical function. Regression analysis may reveal whether there is an essential correlation between the independent and dependent variables and the effect intensity – with what probability the dependent variable can be predicted based on the provided set of independent variables.
About Neural Networks
Artificial Neural Networks (ANN) consist of basic elements known as neurons. The neurons can analyze complex questions, imitate almost all functions, even extremely complex, and provide detailed responses (finding the similarities) based on the set of data. There are three layers of neurons in a neural network: an input layer, the hidden layer, and an output layer. There is more than one secret layer in a Deep Network (DNN), which improves the model’s sophistication and can significantly increase the forecast accuracy.
Neural Networks and Regression
The neural networks can help with the regression analysis — some form of regression model may be turned with the help of a neural network.
As trained, this neural network will descend steadily to find better and more fitting coefficients for the results until the optimal linear return coefficients arrive (or, in neural network terms, the optimal weights for the model).
Revolution of real-life neural networks
Standard regression functions are usually coded in R or Python. To run a neural network model that works similarly to a regression function, a deep learning algorithm can be set with the help of such frameworks or platforms as TensorFlow, CreateML, Google’s Cloud AutoML. As we suggested in the post, while neural networks are feasible and a little harder to grasp, they provide more precise regression results. The more complicated the case, the more benefit you get from “cross the Rubicon” to the realm of fundamental research. It is highly odd to have a regression equation that blends in well with all planned data sets. If you start studying thoroughly, you find that the structures do all the work for you. Specify parameters, and your neural network can build up, execute your tests, and yield results. If you wish to find out more details about the neural networks, check this post, I was impressed by the clearness and digitalization of the explanation there.
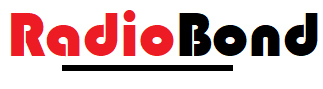